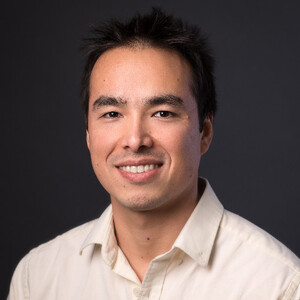
AMA: LinkedIn Vice President of Global Sales Operations, SaaS, Akira Mamizuka on RevOps Reporting
March 26 @ 10:00AM PST
View AMA Answers
Akira Mamizuka
LinkedIn Vice President of Global Sales Operations, SaaS • March 27
We’re on a massive digital transformation journey at LinkedIn. We’re moving off legacy platforms and onto Azure, starting with core data and reporting tools, such as Power BI, Power Apps, and Power Automate. Together, these tools provide significant advantages for LinkedIn, such as: * Firstly, Security → Member Security is our #1 priority and leveraging a platform that prioritizes security (reference) is a must for LinkedIn. * Secondly, Business Agility → Power Apps enables LinkedIn to increase its metabolic rate and unlock Business Agility via rapid-iteration and low-cost application deployments. This strategic move is already paying off as we are in the process of in-housing 3P software that had a high TCO (cost of licensing, maintenance, and personnel) * Lastly, Accessibility and Data Democratization → Azure’s cloud tools make it easy to democratize data and scale with technology. For example, we recently launched a dashboard ecosystem called SaaS KPI that serves as the one-stop-shop for front-line sellers all the way to VP-level executive staff, that places high fidelity insights, with the right level of granularity directly into the hands of decision makers. Together, Power BI, Power Apps, and Power Automate have served as a major unlock for our business and are actively serving our entire sales field along with the broader Revenue Operations functions at LinkedIn.
...Read More409 Views
1 request
Akira Mamizuka
LinkedIn Vice President of Global Sales Operations, SaaS • March 27
One of our first steps was to execute a comprehensive analysis of Data Quality issues, starting with our business use-cases. We then benchmarked our current state against industry standards, such as the DAMA framework. This highlighted opportunities for LinkedIn to improve both over the short-term and the long term, along with improving our data quality rules, such as reducing both false positives and false negatives. Of that effort, 3 items popped stood out: 1. Latency Improvements - We remedied our 2-3 days of latency and the information wasn’t actionable for our operations - this was remedied via dedicated effort from Revenue Operations’ technical teams along with engineering. 2. Erroneous Records & Incomplete Data Sets - On the surface, this might seem like an easy fix, but like most things the devil was in the details. Our data quality engine at the time did not detect issues that were masked in aggregate, so we added rules at deeper levels of granularity. 3. Sustainability → We also hired a Triaging team to maintain and evolve our ecosystem. Data Quality rules are great, but to build a solution that lasts, it’s also important to implement rules at the systems of record to prevent the creation of bad data.
...Read More391 Views
1 request
Akira Mamizuka
LinkedIn Vice President of Global Sales Operations, SaaS • March 27
At LinkedIn, for both target setting and execution purposes, within our SaaS businesses we break down the revenue funnel into discrete parts, each of them mapped to specific teams who are accountable for the results. At the highest level, the first break-down is between “New Business” and “Existing Customers”. For example, within “New Business”, we have a further break-down by “Lead Generation” (owned by Marketing), “Opportunity Creation” (owned by Sales Development and Sales) and “Opportunities Won” (owned by Sales). For each of these parts of the funnel, there are a set of KPIs and targets associated with them. We have dashboards to track performance against each set of KPIs. For example, within “Opportunities Won”, the main KPIs are “Win Rate”, “ASP – Average Selling Price”, and “Average Deal Cycle”. Structuring our reporting in such a way enables us to identify areas of strength and softness during our forecasting process, allowing us to understand the root cause, both in terms of the part the funnel it comes from but also the “cause-effect” relationship between input and output metrics. This structure not only leads to a more accurate forecast, but also allows us to quickly enact action plans with the accountable teams.
...Read More378 Views
1 request
Akira Mamizuka
LinkedIn Vice President of Global Sales Operations, SaaS • March 27
The “bottom-up” process (i.e. the sales teams’ forecast rollup) gives real time sentiment from customers and the field but can be biased by human-led judgment. The “top-down” process (i.e. analyses of consolidated data) brings objectivity and separates signal from noise, though it ignores information that is not yet captured in the data (e.g. a large deal that will be pushed to the next quarter). Over time, I found that a combination of “bottom-up” forecasting with “top-down” forecasting is the most effective way to forecast accurately. A nascent area of capability building and exploration is around using AI and machine learning for forecasting. Collectively, thousands of hours are spent every week in forecasting activities, across all levels of the organization. In the near future, technology will free-up a large portion of this time, at the same time that will make our forecasting and planning processes even more accurate.
...Read More361 Views
1 request
Akira Mamizuka
LinkedIn Vice President of Global Sales Operations, SaaS • March 27
Dashboard proliferation and staleness is an issue companies often deal with, including LinkedIn. Bias to action leads to multiple different dashboards being built over time, leading to: * Inconsistent metrics, since not always the builders align with metric owners on the same source of truth or a consistent way to calculate metrics * Staleness, with dashboards not being maintained to accurately reflect changes in the business * Confusion, with users not knowing which dashboard or report they should refer to To avoid these issues, I’d encourage dashboard builders to ask themselves the following questions before building a new asset: * What is the problem I am solving for? Who is the audience, and what are the key set of metrics needed? * Do we need a new dashboard, or can this use case be solved by augmenting an existing, well-established one? Minimizing the number of surfaces that users interact with is key * What is the established source of truth for the data needed by the dashboard? Who owns the metrics that will be displayed in the dashboard, and how to correctly calculate them? * Who will be responsible for maintaining the dashboard on a recurring basis? Additionally, from time to time it is helpful to do a “clean-up”. For example, remove any dashboards that show limited use in the last 90 days. Also, try to stop sending that weekly report to see how many recipients will reach out asking about it.
...Read More1074 Views
1 request