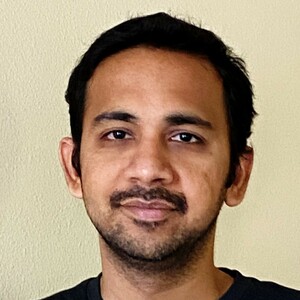
AMA: DocuSign Senior Director of Product, AI/ML Platform, Deepak Mukunthu on AI Product Management
June 28 @ 10:00AM PST
View AMA Answers
Deepak Mukunthu
Salesforce Senior Director of Product, Generative AI Platform (Einstein GPT) • June 28
Assuming you are specifically interested in AI product management, I would suggest these approaches to get started with ML. While I was new to ML/AI, these approaches helped me. 1. Online courses 2. Part-time certifications 3. Conferences 4. Kaggle 5. Publish your work 6. Internship/Volunteering I covered this in my session on AI/ML Product Management: https://www.linkedin.com/video/event/urn:li:ugcPost:6929801568753971200/ If you are looking for guidance on general product management, let me know. There is a lot of material online and I can point you to.
...Read More1192 Views
3 requests
Deepak Mukunthu
Salesforce Senior Director of Product, Generative AI Platform (Einstein GPT) • June 28
AI PMs are expected to have a broad understanding of ML lifecycle and knowledge of key ML trends in the industry. Ensure your product management basics are strong: Deep focus on customers, business priority, foster an experimentation culture. In this session, I also covered key traits of successful AI product managers: https://www.linkedin.com/video/event/urn:li:ugcPost:6929801568753971200/. Hope this helps!
...Read More458 Views
3 requests
Deepak Mukunthu
Salesforce Senior Director of Product, Generative AI Platform (Einstein GPT) • June 28
While working on ML product/feature, there are 2 sets of metrics: 1. Product success metrics that product managers define. Purpose of the is to measure the business/product outcome you are trying to optimize for. Your standard metrics like customer adoption, usage, retention, satisfaction etc. fall in this bucket. So, product managers choose what's best for the situation at hand. 2. ML metrics that data scienstics define. These metrics measure how good the ML approach/solution/model is. For e.g., good metrics to measure a Regression task are: Root Mean Squared Error (RMSE) or Mean Absolute Error (MAE). Product manages and Data scientists collaborate to ensure that the business/product scenario is mapped to ML problem correctly and that there is clear line of site to achieve the product success metrics.
...Read More424 Views
3 requests
Deepak Mukunthu
Salesforce Senior Director of Product, Generative AI Platform (Einstein GPT) • June 28
Broad understanding of ML lifecycle and knowledge of key ML trends in the industry are required to be successful in AI product management role. Beyond this, the level of hard/technical skills required depends on the type of AI product management role - there are 4 different types: 1. Product focused: Infuse intelligence into products (e.g., search, personalization) 2. Platform focused: Infrastructure & tooling for data scientists and ML engineers to manager ML lifecycle 3. Business/Operations focused: ML to improve product adoption/operations (e.g., customer churn prediction) or business outcomes (e.g., revenue forecasting). 4. Research focused: Bringing AI research breakthroughs to market If you are interested in learning more, watch my session on AI/ML Product Management: https://www.linkedin.com/video/event/urn:li:ugcPost:6929801568753971200/
...Read More527 Views
3 requests
Deepak Mukunthu
Salesforce Senior Director of Product, Generative AI Platform (Einstein GPT) • June 28
While infusing ML into a product can be extremely powerful to light-up new scenarios for customers and to optimize current scenarios, it can take a lot of effort to get it right. It all starts with defining and prioritizing the right scenario/problem to focus on, working with data science and ML engineering teams to map the business problem to an ML problem, trying out to different approaches to identify the right one to go ahead with, experimenting and optimizing and then operationalizing the approach and shipping it as part of product stack working with ML engineering and development teams. In addition to focusing on functional aspects and non-functional aspects like performance/scale/reliability, ML scenarios bring in an additional critical aspect of transparency and fairness which is broadly classified under Responsible AI - this is required to gain customer trust and hence feature adoption. It is the responsibility of product manager to define the business scenarios and success metrics to focus on, work closely with data science teams to map that into ML problem and enable them with right data for them to come up with right approach. Then work with ML engineers and developers to operationalize it as part of the product. In summary, it take collaboration between multiple teams/roles in order to ship great ML features as part of a product. If you are interested in learning more, watch my session on AI/ML Product Management: https://www.linkedin.com/video/event/urn:li:ugcPost:6929801568753971200/
...Read More876 Views
4 requests
What are the different types of AI Product Managers. Are we going to see a role that's similar to a Technical Product Manager focused towards the data science team?
My question is more to understand if a PM needs to understand the AI concepts to be a successful AI PM?
Deepak Mukunthu
Salesforce Senior Director of Product, Generative AI Platform (Einstein GPT) • June 28
I have seen 4 different types of AI Product Managers: 1. Product focused: Infuse intelligence into products (e.g., search, personalization) 2. Platform focused: Infrastructure & tooling for data scientists and ML engineers to manager ML lifecycle 3. Business/Operations focused: ML to improve product adoption/operations (e.g., customer churn prediction) or business outcomes (e.g., revenue forecasting). This role typically works with data science teams that work on internal optimizations leveraging ML. 4. Research focused: Bringing AI research breakthroughs to market If you are interested in learning more, watch my session on AI/ML Product Management: https://www.linkedin.com/video/event/urn:li:ugcPost:6929801568753971200/
...Read More492 Views
3 requests