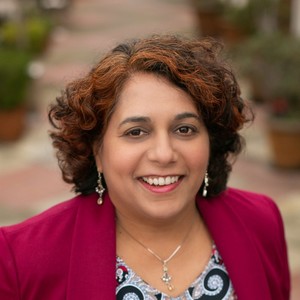
AMA: Cisco Director of Product Management, Speech and Video AI, Savita Kini on AI Product Management
March 3 @ 10:00AM PST
View AMA Answers
What are the different types of AI Product Managers. Are we going to see a role that's similar to a Technical Product Manager focused towards the data science team?
My question is more to understand if a PM needs to understand the AI concepts to be a successful AI PM?
Savita Kini
Cisco Director of Product Management, Speech and Video AI • March 3
AI/ML by definition requires a decent foundational understanding for AI/ML/Deep Learning Concepts, trends in industry, tools and methodologies to be able to work with engineering in defining solutions to customer /user problems. For the forseeable future, I would say that most AI/ML product managers would likely function as both technical as well as regular product managers to blend user journeys into concrete AL/ML solutions.
...Read More602 Views
1 request
Savita Kini
Cisco Director of Product Management, Speech and Video AI • March 3
Key traits for AI PM is no different from other PM roles -- empathy for customer issues, ability craft / create / articulate problems and how we might approach the solution, industry and domain experience, and collaborative leadership to work with engineering. Willingness to learn or prior experience or understanding of AI/ML modeling challenges, and how they can be use in the context the industry / domain where it is applied is ofcourse a big plus.
...Read More651 Views
1 request
Savita Kini
Cisco Director of Product Management, Speech and Video AI • March 3
Metrics are an interesting question. This really depends on the type of product we are building that leverages ML. Since ML can be use for example in electronic records, sales workflows, computer vision type use cases or speech / audio use cases some of which I am familiar with -- we can break it down to product use itself and then algorithm/model used, how often it is used, what kind of business or customer experience metrics it provided or influenced. So the long and short answer is there is no "generic metric" -- we are still building product and features. When it comes to the model itself -- there is the accuracy of the model. Here too -- there is no 100% accuracy, because it is either classifying, predicting, using regressions and so on. There could be again "domain specific" metrics that apply for example for computer vision models. If the model is for example of predicting system failures --- there would be model accuracy metrics to track. We are really getting into "model performance metrics" here.
...Read More374 Views
Savita Kini
Cisco Director of Product Management, Speech and Video AI • May 10
There is no one "metric" that ML Product Teams will use to define success. It entirely depends on what is the "ML" used in the context of a feature or product. Typical metrics might include * Quality improvements achieved via ML implementation vs traditional algorithmic implementations * Usage adoption of AI/ML based feature * Business or user perception metrics of improvements - CSAT, NPS scores * Time savings, $$ savings, etc typical Business metrics. * Benchmarking vs peer products * Accuracy and responsible AI metrics
...Read More383 Views
1 request
Savita Kini
Cisco Director of Product Management, Speech and Video AI • March 3
Seek to understand and clarify first before assuming you have learnt everything that is to know and propose solutions. I see there are lot of online courses, documentation, articles -- you can do a lot of reading online to educate yourself about the complexities of model development, data gathering, data labeling, training and testing. One of the big challenges for AI/ML PMs is understanding whether we have enough data for training the model, is it diverse enough to cover for the specific use-case, model deployment, and corner cases where the model might fail. There is this notion that a few buzz words will help you to convince engineering, please refrain from doing that. If something looks too easy, remember it is not. Ask lot of questions in the early learning phase, no question is dumb. Make sure you have looked at the problem from all different angles, have understood the customer problem, sought out peer perspectives through industry analysts and experts, competitive plays -- so you can define the scope of the solution. With AI/ML and with any other product, perfect is the enemy of the good, but at the sametime, if you have biases in your dataset, the "good enough" might be a disaster as soon as it lands in the hands of your users and customers.
...Read More863 Views
1 request
Savita Kini
Cisco Director of Product Management, Speech and Video AI • March 3
This is not a simple question. There is no such thing as a "traditional PM" because PM roles and responsibilities differ by industry segment. There are substantial differences in PM roles from consumer app to enterprise app to hardware and enterprise infrastructure. Fundamentally the role of the PM is still the same - to consider customer problems, potential solution approaches, and lead them from concept to launch and facilitate a continous innovation lifecycle. The only difference here in AI/ML PM is a new approch to solving the problem with AI/ML tools and frameworks. So anyone with a "Learning mindset", ability to pick up on the AI/ML technologies and comfortable in ambiguity as this field is still growing, -- would do very well.
...Read More762 Views
1 request
Savita Kini
Cisco Director of Product Management, Speech and Video AI • March 3
Excellent question. Hard/technical skills are absolutely critical to be a successful AI product manager. As I mention in my other answers -- PMs bring domain knowledge, and customer perspectives to seek solutions. In AI/ML product development, we apply all the same foundational technical knowledge, with the added AI/ML component. AI/ML models do come at a cost for the compute, so one has to consider optimizations. For example, consider aspects like latency .."how much time will it take to complete this task" , "what is the impact on the user experience or the business workflow". In our Speech Enhancement technology in Webex -- we have to consider improvements in speech quality after extracting noise, background speech, music etc and then package these models into small form factor software libraries to run on the end devices like laptops, mobile phones, desk cameras. In the case of a Cloud/SaaS based product, you are not bound by the constraints of the edge device, since you could run your solution as a microservice in the cloud. The depth and breath of technical skills and ability to keep learning and building your knowledge base is critical for your sucess. We are still in very early stages of this technology shift as it continues to proliferate in every area of our life.
...Read More720 Views
1 request
Savita Kini
Cisco Director of Product Management, Speech and Video AI • March 3
A strong software engineering and product development background is a good foundation to transition to a PM role. AI is a very broad field, and one can apply AI in many different applications and use-cases. Just as you would with any job search and transition -- take some foundational courses in AI/ML so you understand the basics of model development, data issues, ethical/responsible AI. There are many courses online, and you don't have to do actual coding, but since you are a software engineer, might as well get your hands dirty. If you are currently a software engineer, transitioning to a PM role is always harder to do externally. It is easier to do a lateral transition within your own company / organization. Speak to your peers or senior PM leaders to identify opportunity for a stretch assignment or a formal transition. Taking courses offered by plenty of folks like Berkeley extension -- would help you frame your thinking and preparing for a PM Role. Merge the two above to focus on a particular vertical or industry application / SW -- where you can bring domain knowledge. Domain knowledge is super critical as a PM so you can bring the outside in industry, customer, technical skills and experiences together to help define the new features, understand the productization challenges in AI and help the engineering team to build successful products that customers will love.
...Read More985 Views
1 request